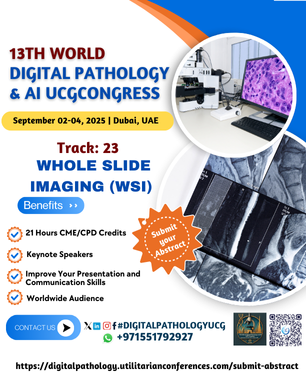
Sub track:-
Enhanced Image Quality Quantitative Analysis, Faster Turnaround Times,...
Sub track:-
Integration of Imaging Modalities, Advanced Image...
Track Overview:
Whole Slide Imaging (WSI) is a pivotal technology
in digital pathology, allowing for the high-resolution scanning of entire
tissue slides and converting them into digital images. This track will explore
the technology behind WSI, its applications in clinical diagnostics and
research, the benefits it offers in terms of workflow efficiency, and the
challenges that come with its implementation. Attendees will gain insights into
how WSI is revolutionizing pathology practice and how it integrates with other
digital pathology technologies, including AI and machine learning.
Key Topics:
Introduction to Whole Slide Imaging: Understanding
the fundamentals of WSI technology, including the scanning process, types of
scanners, and the digital formats used for storing and analyzing slides.
WSI in Clinical Diagnostics: How WSI is enhancing
the accuracy and efficiency of clinical diagnoses, from routine screening to
complex cases such as cancer pathology.
Integration of AI with WSI: The role of artificial
intelligence in enhancing WSI, from automated image analysis and tumor
detection to workflow automation and decision support.
Impact on Pathology Workflows: The benefits and
challenges of integrating WSI into clinical labs, including time savings,
remote consultations, and collaboration among pathologists.
WSI in Education and Training: How WSI is
transforming pathology education by allowing for remote learning, virtual slide
libraries, and interactive case studies for training purposes.
Regulatory and Technical Considerations: Addressing
the regulatory, technical, and quality control issues related to the use of WSI
in clinical practice, including image storage, data security, and validation.
Future Directions of WSI: Emerging trends in WSI
technology, such as higher resolution imaging, multispectral imaging, and
integration with other technologies like molecular pathology and genomics.
Learning Objectives:
Gain an understanding of the technology behind
Whole Slide Imaging (WSI) and its role in digital pathology.
Learn how WSI is being used to improve diagnostic
accuracy and efficiency, particularly in cancer diagnostics and specialized
fields.
Explore the integration of AI with WSI to automate
image analysis and support decision-making in pathology.
Understand the challenges and opportunities that
come with adopting WSI in clinical workflows, including technical, regulatory,
and practical considerations.
Discover how WSI is advancing pathology education,
offering new opportunities for remote learning and virtual slide analysis.
Stay informed about the latest trends in WSI
technology and its potential future developments.
Target Audience:
Pathologists and laboratory professionals
interested in implementing or optimizing WSI in clinical practice.
Researchers exploring the applications of WSI in
clinical and experimental pathology.
Medical educators and trainers looking to leverage
WSI for educational purposes.
Technology developers and vendors in the field of
digital pathology, particularly in WSI technology and AI integration.
Healthcare administrators and policymakers
considering the adoption of WSI in pathology labs.
Speakers/Presenters:
Experts in whole slide imaging technology,
including developers and engineers of WSI systems.
Pathologists who are utilizing WSI in their
diagnostic practice, particularly in oncology, hematology, and other specialty
areas.
AI specialists working on the integration of
artificial intelligence with WSI for automated analysis and decision support.
Healthcare administrators and laboratory managers
discussing the implementation of WSI in clinical workflows.
Regulatory experts addressing the compliance and
validation processes for WSI in clinical settings.
Conclusion:
This track will provide a comprehensive overview of Whole Slide Imaging (WSI), its transformative role in digital pathology, and its applications in both clinical diagnostics and education. Attendees will learn how WSI enhances diagnostic workflows, supports AI-driven analysis, and facilitates remote collaboration. The session will also address key challenges and considerations in adopting WSI technology and explore its future potential in the field of pathology.